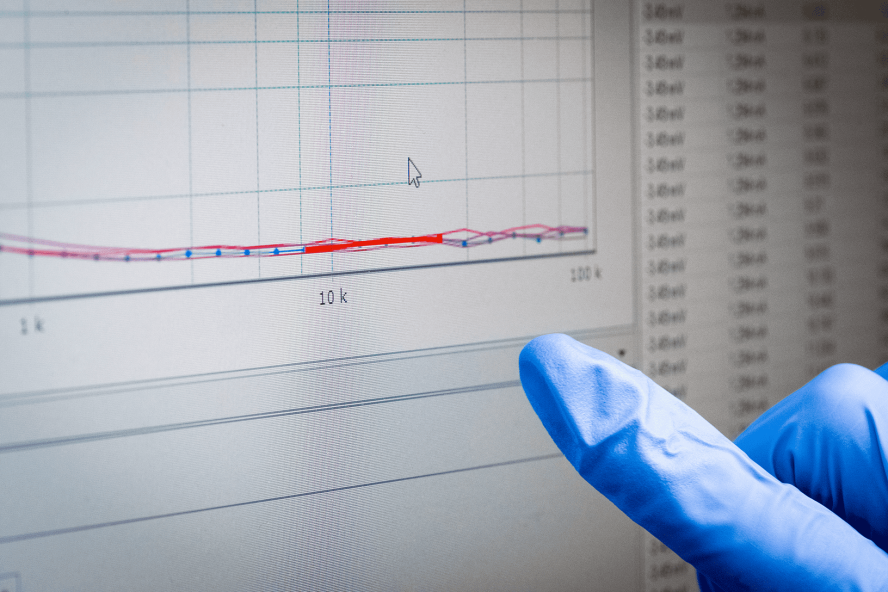
Tufts Institute of Artificial Intelligence (TIAI) facilitates research collaborations across all 10 schools at Tufts University. We have received a number of grants from federal, state, and external funding sources.
Our efforts bring together together faculty, industry experts, and students to advance AI research and development.
Please explore the grants listed below:
2024
ONR: Effective Novelty-Based Human-Machine Interactions in Open-World Outdoor ISR Domains
Principle Investigator: Matthias Scheutz, Karol Family Applied Technology Professor
The overarching goal of this effort is to reach an unprecedented level of open-world novelty characterization, communication, and adaptation that (1) significantly improves open-world situational awareness and understanding in artificial systems, (2) enables effective human- like communications in open-world settings, and (3) allows agents to quickly adapt their courses of action in the interest of meeting their mission objectives.
AFOSR: Learning and Evaluating Explicit Norms in Human-Machine Teaming Contexts and their Impact on Human Trust
Principle Investigator: Matthias Scheutz, Karol Family Applied Technology Professor
The three overarching objectives of this project are (1) to develop comprehensive formalisms for norms that can represent typical norms used in teaming contexts, together with formal model checking approaches that enable norm compliance checks for a given domain model, (2) to systematically compare “implicit” and “explicit” approaches to norm learning and norm following in simulated single and multi-agent domains with and without human actors, and (3) to determine for the best explicit norm-learning and norm-following approach in human- machine contexts whether artificial agents using it to learn and reason with norms meet human expectations of norm-compliant behavior in different teaming tasks.
DARPA: AIE Friction for Accountability in Conversational Transaction (FACT)
Principle Investigators: Research Assistant Professor Vasanth Sarathy and Karol Family Applied Technology Professor Matthias Scheutz
Current AI systems deployed in complex problem-solving and planning scenarios are standalone programs requiring expert authoring of abstract domain representations. The addition of LLMs as front-ends to these systems enables fluency and reduces friction, but also decreases accountability and potentially engendering overtrust. Broadly, what is missing from these approaches is a conceptual framework for “epistemic vigilance”. We propose using argumentation theory to supply that missing conceptual framework for conversational shaping, specifically representing the chat history as argumentation graphs, which describe relationships between premises, conclusions, and evidence expressed by the interlocutors, and anchored to the domain of discourse. This approach will push the brakes on the conversation to promote critical thinking and epistemic vigilance and prevent overtrust.
DARPA (subcontract to Riverside Research): TEAM-PRO: Teaming Evaluation and Assessment Methodologies for Performance- and Readiness-prediction Objectively
Principle Investigator: Matthias Scheutz, Karol Family Applied Technology Professor
TEAM PRO addresses key challenges of team performance prediction by incorporating multi-dimensional candidate signatures from cognitive, interpersonal, and physiological domains, reflecting the diverse nature of team dynamics. We tackle the challenge of sparse data, particularly limited ground-truth labels, by augmenting it with simulation data of a teaming scenario like FiST training. This approach generates data motifs in staged scenarios that reveal key teaming challenges, such as communication failures, aiding in identifying predictive signatures. Our use of supplemental Human Subject Research further enables generalization across the program. Employing Large Language Models (LLMs) for automated analysis of team communications provides deep insights and enhanced analytical capabilities, crucial for understanding team interactions, task events, and conversational dynamics.